Nachdem die Maus gerne mal vorbei schaut und die Nahrung stibitzt, hab ich mir etwas überlegt, wie man das verhindern könnte.
Thema 1: das Vertreiben
Lebend-Mausefalle: scheidet aus, weil zu viel Arbeit damit verbunden ist.
Kleiner Stromschlag: scheidet aus, weil „wenig“ Strom vorhanden ist durch die PoE Architektur. Außerdem ein wenig brachial.
Also habe ich einen 5 Volt Motor mit 14.000 Umdrehungen ins Gehäuse eingebaut – der rattert und vibriert anständig und scheint den Job des Erschreckens gut zu erledigen:
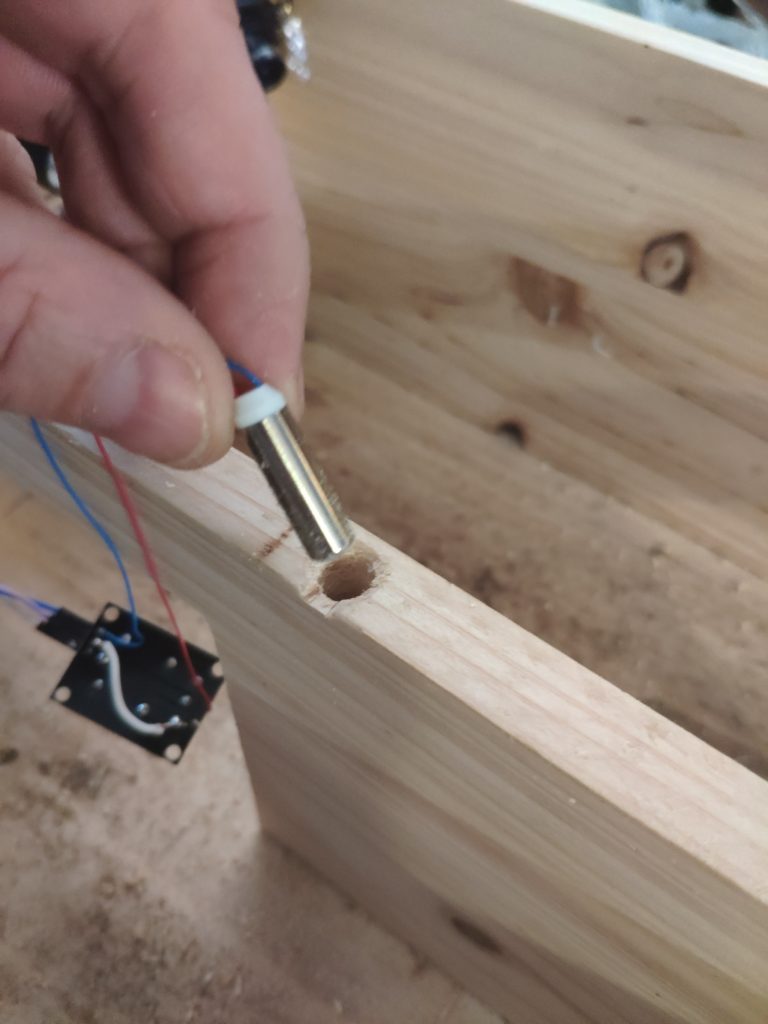
Thema 2: das Erkennen
Natürlich soll spezifisch nur alles vertrieben werden was KEIN Igel ist: Also Katzen, Mäuse, Schlangen, Krokodile etc.
Derzeit im Testlauf wird nur über eine normale Bewegungserkennung („Pixeländerung“) getriggert – und nur tagsüber um nicht versehentlich einen Igel zu schrecken.
Für den echten Betrieb habe ich aber schon eine KI anhand eines neuronalen Netzes (genauer: ein Convolutional Neural Network) gebaut und mit den Bildern der Kamera spezifisch trainiert. Hier die Struktur des Netzes:
_________________________________________________________________
Layer (type) Output Shape Param #
=================================================================
input_2 (InputLayer) [(None, 224, 224, 3)] 0
_________________________________________________________________
conv1_pad (ZeroPadding2D) (None, 225, 225, 3) 0
_________________________________________________________________
conv1 (Conv2D) (None, 112, 112, 32) 864
_________________________________________________________________
conv1_bn (BatchNormalization (None, 112, 112, 32) 128
_________________________________________________________________
conv1_relu (ReLU) (None, 112, 112, 32) 0
_________________________________________________________________
conv_dw_1 (DepthwiseConv2D) (None, 112, 112, 32) 288
_________________________________________________________________
conv_dw_1_bn (BatchNormaliza (None, 112, 112, 32) 128
_________________________________________________________________
conv_dw_1_relu (ReLU) (None, 112, 112, 32) 0
_________________________________________________________________
conv_pw_1 (Conv2D) (None, 112, 112, 64) 2048
_________________________________________________________________
conv_pw_1_bn (BatchNormaliza (None, 112, 112, 64) 256
_________________________________________________________________
conv_pw_1_relu (ReLU) (None, 112, 112, 64) 0
_________________________________________________________________
conv_pad_2 (ZeroPadding2D) (None, 113, 113, 64) 0
_________________________________________________________________
conv_dw_2 (DepthwiseConv2D) (None, 56, 56, 64) 576
_________________________________________________________________
conv_dw_2_bn (BatchNormaliza (None, 56, 56, 64) 256
_________________________________________________________________
conv_dw_2_relu (ReLU) (None, 56, 56, 64) 0
_________________________________________________________________
conv_pw_2 (Conv2D) (None, 56, 56, 128) 8192
_________________________________________________________________
conv_pw_2_bn (BatchNormaliza (None, 56, 56, 128) 512
_________________________________________________________________
conv_pw_2_relu (ReLU) (None, 56, 56, 128) 0
_________________________________________________________________
conv_dw_3 (DepthwiseConv2D) (None, 56, 56, 128) 1152
_________________________________________________________________
conv_dw_3_bn (BatchNormaliza (None, 56, 56, 128) 512
_________________________________________________________________
conv_dw_3_relu (ReLU) (None, 56, 56, 128) 0
_________________________________________________________________
conv_pw_3 (Conv2D) (None, 56, 56, 128) 16384
_________________________________________________________________
conv_pw_3_bn (BatchNormaliza (None, 56, 56, 128) 512
_________________________________________________________________
conv_pw_3_relu (ReLU) (None, 56, 56, 128) 0
_________________________________________________________________
conv_pad_4 (ZeroPadding2D) (None, 57, 57, 128) 0
_________________________________________________________________
conv_dw_4 (DepthwiseConv2D) (None, 28, 28, 128) 1152
_________________________________________________________________
conv_dw_4_bn (BatchNormaliza (None, 28, 28, 128) 512
_________________________________________________________________
conv_dw_4_relu (ReLU) (None, 28, 28, 128) 0
_________________________________________________________________
conv_pw_4 (Conv2D) (None, 28, 28, 256) 32768
_________________________________________________________________
conv_pw_4_bn (BatchNormaliza (None, 28, 28, 256) 1024
_________________________________________________________________
conv_pw_4_relu (ReLU) (None, 28, 28, 256) 0
_________________________________________________________________
conv_dw_5 (DepthwiseConv2D) (None, 28, 28, 256) 2304
_________________________________________________________________
conv_dw_5_bn (BatchNormaliza (None, 28, 28, 256) 1024
_________________________________________________________________
conv_dw_5_relu (ReLU) (None, 28, 28, 256) 0
_________________________________________________________________
conv_pw_5 (Conv2D) (None, 28, 28, 256) 65536
_________________________________________________________________
conv_pw_5_bn (BatchNormaliza (None, 28, 28, 256) 1024
_________________________________________________________________
conv_pw_5_relu (ReLU) (None, 28, 28, 256) 0
_________________________________________________________________
conv_pad_6 (ZeroPadding2D) (None, 29, 29, 256) 0
_________________________________________________________________
conv_dw_6 (DepthwiseConv2D) (None, 14, 14, 256) 2304
_________________________________________________________________
conv_dw_6_bn (BatchNormaliza (None, 14, 14, 256) 1024
_________________________________________________________________
conv_dw_6_relu (ReLU) (None, 14, 14, 256) 0
_________________________________________________________________
conv_pw_6 (Conv2D) (None, 14, 14, 512) 131072
_________________________________________________________________
conv_pw_6_bn (BatchNormaliza (None, 14, 14, 512) 2048
_________________________________________________________________
conv_pw_6_relu (ReLU) (None, 14, 14, 512) 0
_________________________________________________________________
conv_dw_7 (DepthwiseConv2D) (None, 14, 14, 512) 4608
_________________________________________________________________
conv_dw_7_bn (BatchNormaliza (None, 14, 14, 512) 2048
_________________________________________________________________
conv_dw_7_relu (ReLU) (None, 14, 14, 512) 0
_________________________________________________________________
conv_pw_7 (Conv2D) (None, 14, 14, 512) 262144
_________________________________________________________________
conv_pw_7_bn (BatchNormaliza (None, 14, 14, 512) 2048
_________________________________________________________________
conv_pw_7_relu (ReLU) (None, 14, 14, 512) 0
_________________________________________________________________
conv_dw_8 (DepthwiseConv2D) (None, 14, 14, 512) 4608
_________________________________________________________________
conv_dw_8_bn (BatchNormaliza (None, 14, 14, 512) 2048
_________________________________________________________________
conv_dw_8_relu (ReLU) (None, 14, 14, 512) 0
_________________________________________________________________
conv_pw_8 (Conv2D) (None, 14, 14, 512) 262144
_________________________________________________________________
conv_pw_8_bn (BatchNormaliza (None, 14, 14, 512) 2048
_________________________________________________________________
conv_pw_8_relu (ReLU) (None, 14, 14, 512) 0
_________________________________________________________________
conv_dw_9 (DepthwiseConv2D) (None, 14, 14, 512) 4608
_________________________________________________________________
conv_dw_9_bn (BatchNormaliza (None, 14, 14, 512) 2048
_________________________________________________________________
conv_dw_9_relu (ReLU) (None, 14, 14, 512) 0
_________________________________________________________________
conv_pw_9 (Conv2D) (None, 14, 14, 512) 262144
_________________________________________________________________
conv_pw_9_bn (BatchNormaliza (None, 14, 14, 512) 2048
_________________________________________________________________
conv_pw_9_relu (ReLU) (None, 14, 14, 512) 0
_________________________________________________________________
conv_dw_10 (DepthwiseConv2D) (None, 14, 14, 512) 4608
_________________________________________________________________
conv_dw_10_bn (BatchNormaliz (None, 14, 14, 512) 2048
_________________________________________________________________
conv_dw_10_relu (ReLU) (None, 14, 14, 512) 0
_________________________________________________________________
conv_pw_10 (Conv2D) (None, 14, 14, 512) 262144
_________________________________________________________________
conv_pw_10_bn (BatchNormaliz (None, 14, 14, 512) 2048
_________________________________________________________________
conv_pw_10_relu (ReLU) (None, 14, 14, 512) 0
_________________________________________________________________
conv_dw_11 (DepthwiseConv2D) (None, 14, 14, 512) 4608
_________________________________________________________________
conv_dw_11_bn (BatchNormaliz (None, 14, 14, 512) 2048
_________________________________________________________________
conv_dw_11_relu (ReLU) (None, 14, 14, 512) 0
_________________________________________________________________
conv_pw_11 (Conv2D) (None, 14, 14, 512) 262144
_________________________________________________________________
conv_pw_11_bn (BatchNormaliz (None, 14, 14, 512) 2048
_________________________________________________________________
conv_pw_11_relu (ReLU) (None, 14, 14, 512) 0
_________________________________________________________________
conv_pad_12 (ZeroPadding2D) (None, 15, 15, 512) 0
_________________________________________________________________
conv_dw_12 (DepthwiseConv2D) (None, 7, 7, 512) 4608
_________________________________________________________________
conv_dw_12_bn (BatchNormaliz (None, 7, 7, 512) 2048
_________________________________________________________________
conv_dw_12_relu (ReLU) (None, 7, 7, 512) 0
_________________________________________________________________
conv_pw_12 (Conv2D) (None, 7, 7, 1024) 524288
_________________________________________________________________
conv_pw_12_bn (BatchNormaliz (None, 7, 7, 1024) 4096
_________________________________________________________________
conv_pw_12_relu (ReLU) (None, 7, 7, 1024) 0
_________________________________________________________________
conv_dw_13 (DepthwiseConv2D) (None, 7, 7, 1024) 9216
_________________________________________________________________
conv_dw_13_bn (BatchNormaliz (None, 7, 7, 1024) 4096
_________________________________________________________________
conv_dw_13_relu (ReLU) (None, 7, 7, 1024) 0
_________________________________________________________________
conv_pw_13 (Conv2D) (None, 7, 7, 1024) 1048576
_________________________________________________________________
conv_pw_13_bn (BatchNormaliz (None, 7, 7, 1024) 4096
_________________________________________________________________
conv_pw_13_relu (ReLU) (None, 7, 7, 1024) 0
_________________________________________________________________
global_average_pooling2d (Gl (None, 1024) 0
_________________________________________________________________
dense_4 (Dense) (None, 3) 3075
=================================================================
Total params: 3,231,939
Trainable params: 1,866,755
Non-trainable params: 1,365,184
Natürlich habe ich die Welt nicht selbst erfunden sondern mittels „transfer learning“ ein bereits sehr gut trainiertes Netz hergenommen, einige Layer entfernt und dafür meine eigenen trainierbaren Layer eingebaut. Während des Trainierens habe ich die Layer des mobilenet-Netzes gesperrt um ein neuerliches Trainieren zu verhindern.
Und tatsächlich konnte ich schon eine 100%ige Trefferquote bei der Klassifikation der Tiere erreichen:
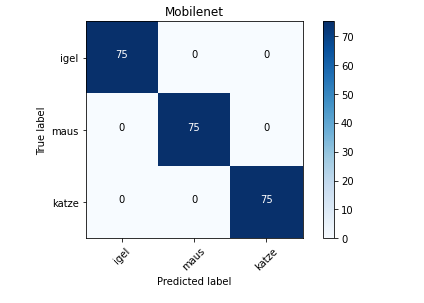
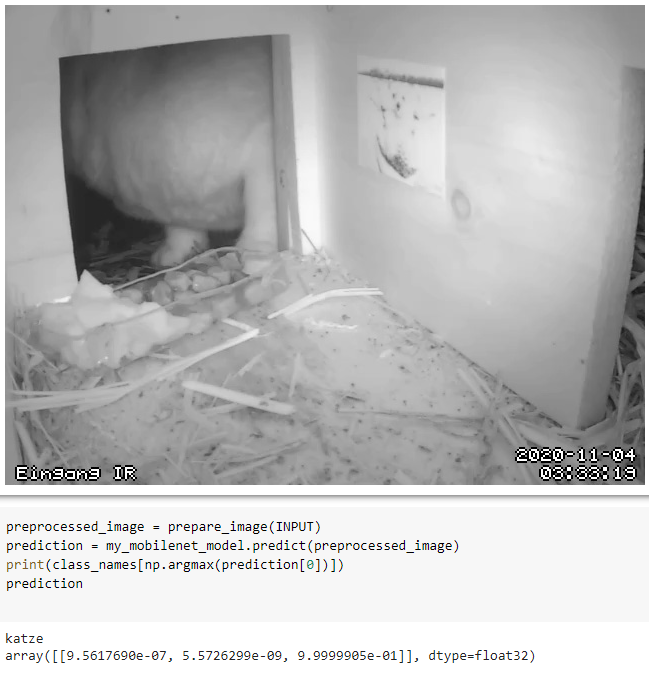
Die Katze wurde in diesem Bild eindeutig gefunden, erkannt und korrekt klassifiziert! Jetzt muss das nur noch in den Workflow eingebaut werden: das Igel-Modell wird gehostet und per REST zur Verfügung gestellt – und dann von der Bewegungserkennung getriggert. Das dürfte noch ein paar Tage dauern – aber ich sehe keine großen Fallstricke mehr.
Ich glaube man darf behaupten, dass dies mittlerweile das intelligenteste Igelheim auf dem Planeten ist 🙂